Dissertation defense (May 17, 2022): Danielle Fontes de Albuquerque
Student: Danielle Fontes de Albuquerque
Title: Feature Selection in Brazilian higher education data
Advisors: Rafaelli Coutinho (advisor) and Diego Brandão (CEFET/RJ) (co-advisor)
Committee: Rafaelli Coutinho (president), Diego Brandão (CEFET/RJ), Eduardo Ogasawara (CEFET/RJ), Alessandro Vivas Andrade (UFVJM), Cristiano Maciel (UFMT)
Day/Time: May 17, 2022 / 2:30 p.m.
Room: https://us06web.zoom.us/j/83229649478?pwd=cm90SXRXM1pDN2duWFlLdXlnRjBkUT09
Abstract: Increasingly, the education sector is using its extensive data repositories to aid decision-making within universities. One of the main problems these institutions face is the dropout of students. It is a worrying phenomenon because it causes social and economic losses for both students and society. One way to reduce the impact of dropouts is to identify the possible causes of dropouts using the databases available in the institutions. Educational Data Mining (EDM) is an interdisciplinary area that uses computational and statistical techniques to understand the educational scenario from the databases of educational institutions. Within this area, Feature Selection (FS) is a set of techniques capable of identifying which are the most relevant attributes in a large database and simplifying it so that it is possible to express the information with a smaller volume of data. With this, it is possible to perform analysis on smaller and cleaner databases, which facilitates the problem understanding and improves computational performance in terms of processing time and the quality of the model generated. Furthermore, identifying the most relevant factors is a way to understand the possible causes and consequences of the problem. This work performs a comparative analysis of FS techniques on educational data from CES, provided by the Brazilian government, which gathers information about all higher education students in the country. The goal is to identify what are the main factors involved in higher education dropout and find combinations of FS techniques and classifiers that enhance the quality of the classification. A new approach for FS was also proposed with a Genetic Algorithm (GA) to allow more flexibility and specificity in the educational setting, called FlexAG. The results show that the attributes year of entry, extracurricular activity, and student financing are the most important for the overall base scenario of CES. In addition, the FS techniques can improve the classification performance measures, and reduce the number of attributes and classification time.
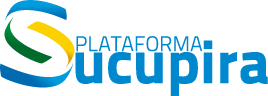