Defesa de dissertação (14/12/2023): Ryan Dutra de Abreu
Discente: Ryan Dutra de Abreu
Título: Um Estudo sobre a Integração de Algoritmos de Filtragem Colaborativa e Detecção de Comunidades para Melhoria do Desempenho das Recomendações
Orientadores: Laura Silva de Assis (orientadora) e Douglas de Oliveira Cardoso (co-orientador)
Banca: Laura Silva de Assis (Cefet/RJ), Douglas de Oliveira Cardoso (IPT), Diego Nunes Brandão (Cefet/RJ) e João Nuno Vinagre Marques da Silva (NESC TEC / Universidade do Porto)
Dia/hora: 14 de dezembro de 2023, às 11h
Resumo: A recomendação de itens em sistemas de recomendação é uma técnica amplamente utilizada para auxiliar os usuários na descoberta de conteúdo relevante. Tradicionalmente, os algoritmos de recomendações baseados em filtragem colaborativa são os mais utilizados no campo devido ao seu bom desempenho frente às demais abordagens. Esses algoritmos buscam identificar padrões globais de similaridade entre usuários ou itens para gerar recomendações. Neste trabalho, investigamos como a combinação das tarefas de recomendação e detecção de comunidades pode levar a recomendações melhores do que aquelas obtidas sem considerar comunidades implícitas. Para isso, avaliamos experimentalmente várias combinações de métodos para detecção de comunidades e algoritmos de recomendação, os quais foram submetido à experimentos computacionais considerando tanto conjuntos de dados sintéticos quanto reais. O objetivo intrínseco deste esforço foi o de revelar padrões interessantes no comportamento dos sistemas resultantes. Os resultados obtidos mostram que a inclusão de detectores de comunidades no sistema pode melhorar significativamente tanto a eficácia quanto a eficiência dos algoritmos de recomendação em alguns cenários. Essas descobertas podem ser usadas para ajudar pesquisadores e profissionais de ciência de dados a compreender melhor os benefícios e limitações dessa metodologia. Por fim, as descobertas aqui obtidas podem ser aplicadas em menor ou maior grau em diversos domínios onde a personalização de recomendações em nível local pode ser uma abordagem eficaz para melhorar a experiência do usuário, sobretudo em situações onde exista uma grande estrutura de redes e comunidades bem definidas.
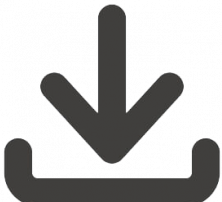