Defesa de dissertação (14/11/2024): Augusto José Moreira da Fonseca
Discente: Augusto José Moreira da Fonseca
Título: Interpolação de Precipitação por Redes Neurais Convolucionais para Grafos
Orientadores: Eduardo Bezerra da Silva (orientador) e Fabio Andre Machado Porto (coorientador)
Banca: Eduardo Bezerra da Silva (Cefet/RJ – PPCIC), Fabio Andre Machado Porto (LNCC), Eduardo Soares Ogasawara (Cefet/RJ – PPCIC), Mariza Ferro (UFF), Leonardo Silva de Lima (UFPR)
Data: 14 de novembro de 2024, às 10h
Resumo: O monitoramento e a previsão das condições climáticas atmosféricas têm implicações significativas em diversos campos, como agricultura, transporte e segurança pública. Previsões eficazes das condições climáticas podem ajudar a mitigar e prevenir os impactos adversos de eventos climáticos severos, incluindo a perda de vidas humanas. Com os avanços tecnológicos, existem vários instrumentos disponíveis para observação atmosférica, como satélites, radares e estações de superfície. Esses instrumentos fornecem observações em tempo real e, em alguns casos com alta resolução espacial e temporal, tornado-se insumo ideal para o treinamento de modelos de previsão do tempo baseados em Aprendizado de Máquina (AM). Por outro lado, um desafio acerca dos dados meteorológicos é a disponibilidade e distribuição espacial das estações de superfície. Por conta de sua distribuição desigual, certas áreas acabam com monitoramento insuficiente. Métodos de interpolação são comumente usados para inferir valores de precipitação para áreas não cobertas. No entanto, esses métodos frequentemente negligenciam o contexto e a física da atmosfera, resultando em discrepâncias entre os dados interpolados e os observados. Além disso, esses métodos não levam em consideração o espaço e o tempo simultaneamente. Este estudo tem como objetivo implementar uma Rede Convolucional Espaço-Temporal de Grafos (STGCN, na sigla em inglês) para interpolar dados de precipitação, que é uma variável significativamente desbalanceada. A STGCN é adaptada para realizar a interpolação da precipitação, aprendendo os padrões e a física da atmosfera em séries temporais multivariadas de múltiplos instrumentos meteorológicos. O objetivo é melhorar a precisão da interpolação, especialmente em áreas com cobertura limitada de estações de superfície. Comparamos os resultados alcançados pela STGCN com os de um método tradicional de interpolação, o Inverse Distance Weighting (IDW). Os resultados alcançados apontam que, comparado ao IDW, a STGCN é capaz de obter melhor acurácia em eventos mais extremos de precipitação e alcançam resultados promissores em eventos de menor magnitude. Em alguns casos, nosso modelo chegou a alcançar uma acurácia para eventos mais extremos entre 40% e 80% maior que o método IDW. No entanto, identificamos que o desbalanceamento dos dados de precipitação e a relativa baixa quantidade de exemplos de eventos extremos podem prejudicar a acurácia da STGCN em alguns cenários. Nosso método se mostra promissor e abre caminho para futuras pesquisas.
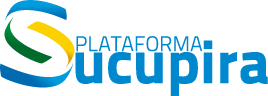
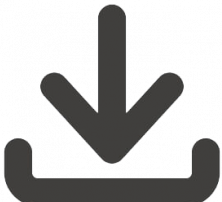