Dissertation defense (December 02, 2024): Leandro de Mattos Bento Soares
Student: Leandro de Mattos Bento Soares
Title: Fall Detect of combat soldiers using artificial neural networks
Advisores: Eduardo Bezerra da Silva (advisor) and Diego Nunes Brandão (co-advisor)
Committee: Eduardo Bezerra da Silva (PPCIC / CEFET-RJ), Diego Nunes Brandão (PPCIC / CEFET-RJ), Pedro Henrique González (PPCIC / CEFET-RJ), Pablo Rangel (IPqM), Cláudio Miceli de Farias (PESC / UFRJ)
Day/Time: December 02, 2024 / 9 a.m
Abstract: During military operations involving combat soldiers, they face a series of physical challenges due to the nature of the activities, including injuries that can result in hypovolemic hemorrhagic shock and, in extreme cases, lead to death. Thus, it is essential that the control centers of these operations be quickly informed about the condition of the combatants, allowing for the acceleration of rescue efforts and the preservation of their lives. In this context, the Brazilian Navy developed the “Future Combatant” project, which aims to create models capable of detecting situations requiring immediate assistance for the combatant, as well as other relevant occurrences, such as conflicts or risk situations, that can be automatically identified from the inertial variations of the combatants. For the collection of these inertial variables, specific devices were chosen: a smartwatch model, positioned on the right and left wrists, and a smartphone model, positioned on the soldier’s chest. Within this project, the investigation of methods for detecting soldier falls stands out, divided into two main stages. The first stage involves implementing a neural network model to detect fall patterns, framing the problem as a classification task. The second stage aims to propose a binary classifier to differentiate operational falls from those caused by injuries. This dissertation focuses on fall detection, belonging to the first stage. For this research, data were collected on daily life activities, fall activities, and military activities with and without carrying weapons from 15 members of the Brazilian Navy, simulating inputs for the neural network model to be implemented and tested in field situations. From this dataset, 12 experimental scenarios were established for each sensor position, explored in the time and frequency domains, aiming to identify the set of inertial variables, sensor positions, and the domain that presented the best performance in classifying fall events. The chosen configuration set was implemented in the CNN1D neural network architecture, whose hyperparameters were adjusted through Bayesian optimization.
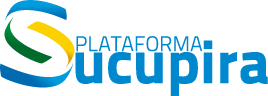
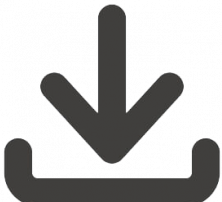